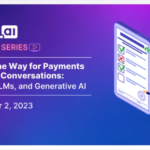
Introduction to Generative AI: Exploring the Basics
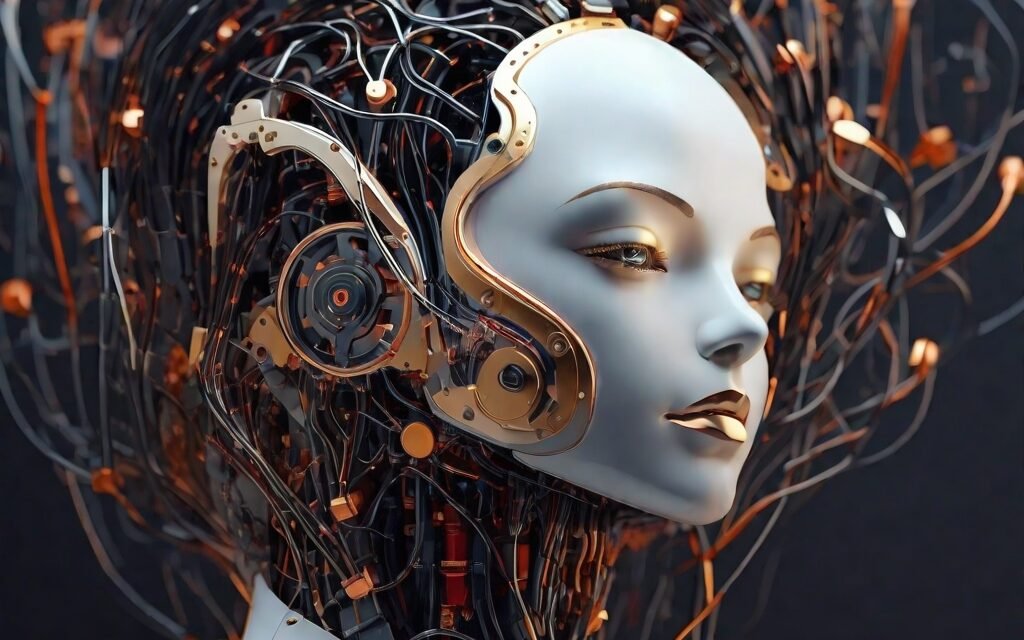
Introduction to Generative AI: Exploring the Basics
Generative Artificial Intelligence (AI) is a rapidly expanding field that is transforming the way we think about intelligent systems. Generative AI focuses on creating machine learning models that can generate new content that is similar to what it was trained on. With its ability to create high-quality, realistic outputs like images, videos, and text, Generative AI is opening up new avenues for creativity, automation, and innovation. In this article, we’ll explore the basics of Generative AI.
Machine Learning Basics
Before diving into Generative AI, it’s crucial to understand the principles of machine learning (ML). Machine learning is a subfield of artificial intelligence that focuses on building models that can learn from data. ML models are trained on input data and use patterns and relationships in the data to make predictions or identify patterns in new data.
Machine learning is typically divided into three categories:
Supervised Learning: The algorithm is trained on labeled data, such as input-output pairs. The goal is to learn a mapping between inputs and outputs.
Unsupervised Learning: The algorithm is trained on unlabeled data and must discover inherent patterns or structure in the data without any prior knowledge of the output.
Reinforcement Learning: The algorithm learns through trial and error by receiving rewards or penalties for certain actions.
What is Generative AI?
Generative AI is a subset of machine learning that focuses on creating models that can generate new content that is similar to what it was trained on. Generative models typically fall into two categories:
Generative Adversarial Networks (GANs): A GAN is a set of two neural networks—one generator and one discriminator—that work together to generate new content. The generator creates output that is fed into the discriminator, which evaluates the output and provides feedback to the generator. The generator then adjusts its output based on the feedback from the discriminator, creating an ongoing feedback loop.
Variational Auto Encoders (VAEs): A VAE is a type of generative model that uses an encoder to compress input data into a latent space and a decoder network to then decode the input data from the compressed latent space. The latent space can be manipulated to produce new, similar outputs.
Applications of Generative AI
Generative AI has numerous applications in various fields, including:
Art and Design: Generative AI can be used to create original artworks, designs, and videos, and to generate new variations of existing media.
Data Augmentation: Generative AI can generate synthetic data that can be used for training machine learning models on limited or imbalanced datasets.
Simulation and Exploration: Generative AI can be used to simulate different scenarios and explore new solutions without the need for real-world data.
Personalization: Generative AI can generate personalized experiences, like product recommendations.
Conclusion
Generative AI is an exciting field with huge potential for innovation and creativity. It is opening up new avenues for data augmentation, personalization, and exploration, but it does come with some ethical, legal, and computational challenges. Understanding the basics of Generative AI is vital to grasp its capabilities and limitations. We hope this introduction has provided you with an overview of Generative AI and its potential applications.