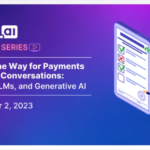
Generative AI & its Pro’s and Con’s
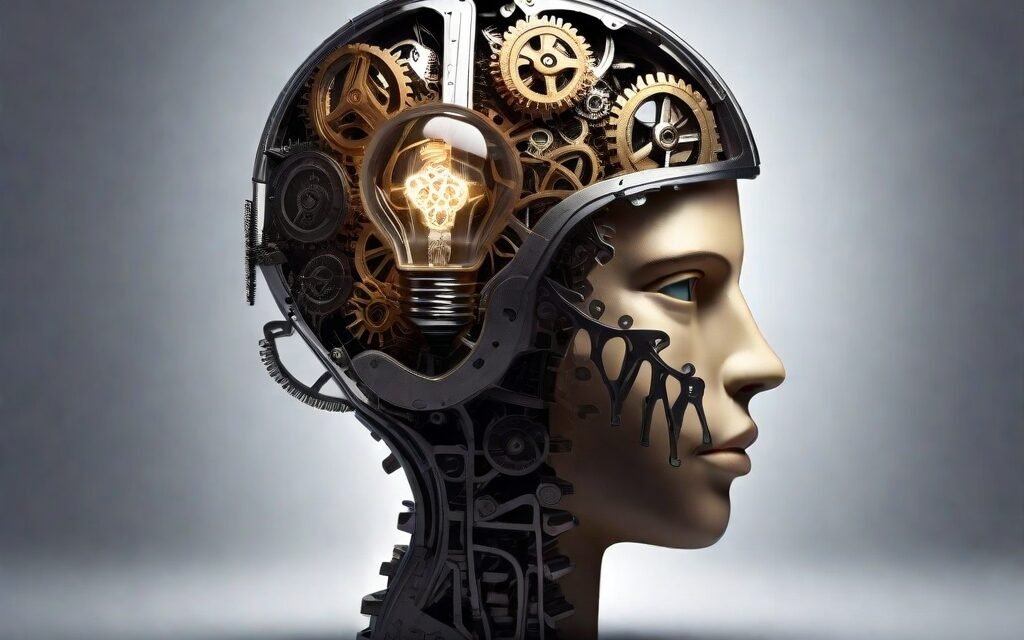
Generative AI & its Pro’s and Con’s
Generative Artificial Intelligence (AI) is a subset of machine learning that focuses on creating models that can generate new content, such as images, videos, text, and even music, that is similar to what it was trained on. It has gained a lot of attention in recent years due to its ability to produce realistic and creative outputs. In this blog post, we’ll explore the pros and cons of Generative AI.
Pros of Generative AI:
- Creative Potential: Generative AI models have the potential to create original and unique content, making them an excellent tool for artists, designers, and creatives looking for new sources of inspiration.
- Data Augmentation: By generating synthetic data that mimics real data, Generative AI can help augment training datasets, leading to improved model performance and generalization. This is particularly useful when working with limited or imbalanced datasets.
- Efficiency: Generative AI techniques, such as generative adversarial networks (GANs), can generate high-quality outputs efficiently. For example, GANs can create high-resolution images with impressive realism, saving time and effort compared to manual creation.
- Exploration and Simulation: Generative AI can be used to simulate and explore different scenarios. For example, in healthcare, GANs can generate synthetic medical images for training models or testing treatment strategies without the need for an extensive collection of real patient data.
- Personalization: Generative AI models can be used to create personalized recommendations and experiences. For instance, they can be applied in e-commerce to generate personalized product recommendations or in marketing to create targeted advertisements.
Cons of Generative AI:
- Ethical Concerns: The ability of Generative AI to create realistic content raises ethical concerns, including the potential for misuse or the creation of deepfake content that can be used for malicious purposes, such as spreading misinformation or identity theft.
- Data Bias: Generative AI models heavily rely on the data they are trained on. If the training data is biased or lacks diversity, the generated outputs may also inherit these biases. Steps must be taken to ensure fairness and inclusiveness in the training data and the generated outputs.
- Lack of Control: Generative AI models often operate in a black box manner, making it challenging to understand how they arrive at their generated outputs. This lack of control can be unsettling, particularly in critical applications like healthcare or autonomous systems where explainability is crucial.
- Computational Requirements: Training and running large-scale Generative AI models can be computationally intensive, requiring powerful hardware and substantial computational resources. This can limit accessibility and practicality for many users, especially those with limited budgets or access to infrastructure.
- Intellectual Property: The question of who owns the generated content and how it can be used is still a subject of legal debate. As Generative AI becomes more prevalent, clarifying the IP rights and regulations around generated content will become increasingly important.
Generative AI holds significant promise for various applications, from creative endeavors to data augmentation and simulation. However, it is essential to be aware of the challenges it presents, such as ethical considerations, biases, and the need for computational resources. Striking a balance between innovation and responsible use is key to leveraging the potential of Generative AI while mitigating its drawbacks.